Hi tweeps (I know most of you arrive here via Twitter (except for that one US bot who watches the same 10 pages every night (hi you!)))
So I’m sitting in the Tokyo-Sidney plane, which has no power plugs and broken headphones, and I thought, what can I do while waiting to land on the continent with the weirdest fauna in the world?
The answer is, talk about my own computer generated weird species of course. This post is the follow up to this one and this one, and to a lesser extent, this and this. Actually the results have been sitting in my computer since last summer; I posted a bit on twitter too. In short, OEE is about building a living world that is “forever interesting”. Since you’ve got all the theory summed up in the previous posts, let’s go directly to the implementation and results.
To be honest, I don’t remember all the details exactly. But here we go!
Here is what the 1st batch looked like, at the very beginning of the simulation:
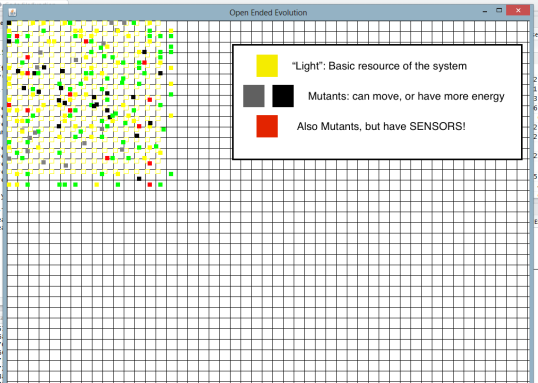
So you have an artificial world with individuals that we hope will evolve into something interesting through interactions with each other. The yellow individuals are how we input free energy in the system. It means that they appear every few steps, “out of nothing”. They cannot move, or eat, or do anything interesting really. They just contain energy, and when the energy is used up, they die. I call it “light”.
Then you have the mutants, which appear on the field like light, but with a random twist. Maybe they can move, or hypothetically store more energy. Mutants can produce one or more kids if they reach a given energy threshold (they do not need a mate to reproduce.) The kid can in turn be copy of their parents, or mutants of mutants.
Then you have the interesting mutants. These have sensors: if something has properties that their sensors can detect, they eat it (or try to). Eating is the only way to store more energy, which can then be used to move, or have kids, or just not die, so it’s pretty important.
Now remember that this sim is about the Interface Theory of Perception. In this case it means that each sensor can only detect a precise value of a precise property. For example, maybe I have a sensor that can only detect individuals who move at exactly 1 pixel/second. Or another sensor that detects individuals that can store a maximum of 4 units of energy. Or have 2 kids. Or have kids when they reach 3 units of energy. Or give birth to kids with a storage of 1 unit of energy.
A second important point is, you can only eat people who have less energy than you do, otherwise you lose energy or even die. BUT, to make things interesting, there is no sensor allowing you to measure how much energy this other guy has right now.
It sounds a bit like the real world, no? You can say that buffaloes that move slowly are maybe not very energetic, so as a lion, you should try to eat them. But there is no guarantee that you will actually be able to overpower them.
Before we get there, there is a gigantic hurdle. Going from “light” to lions or buffaloes is not easy. You need sensors, but sensors require energy. And mutations appear randomly, so it takes a lot of generations to get the first viable mutant: something that can detect and eat light, but doesn’t eat their own kids by mistake. Here is what the origin of life looks like in that world:
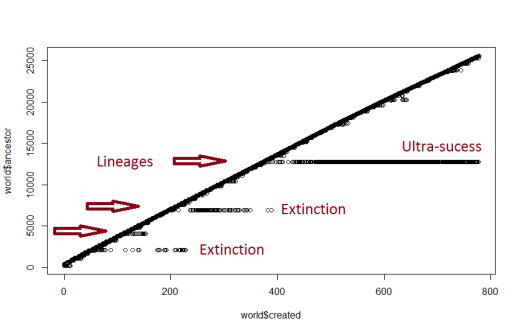
The Y axis is the ID of the oldest ancestor, and X is the time. Everything on the diagonal is just regular light. All the horizontal branches are mutant lineages; as you can see, there are lots of false starts before we get an actual species to get off! Then at a longer timescale this happens:

This is the same sim as before, but zoomed out. Lots of interesting stuff here. First, or previously successful descendants of #14000 go extinct just as a new successful lineage comes in. This is not a coincidence. I think either both species were trying to survive on the same energy source (light) and one starved out the other, or one just ate the other to extinction.
Seeing as the slope of the “light” suddenly decreases as species 2 starts striving, I think the 1st hypothesis is the right one.
Now the fact that our successful individuals all have the same ancestor doesn’t actually mean that they belong to the same species. Actually, this is what the tree of life looks like in my simulated worlds:
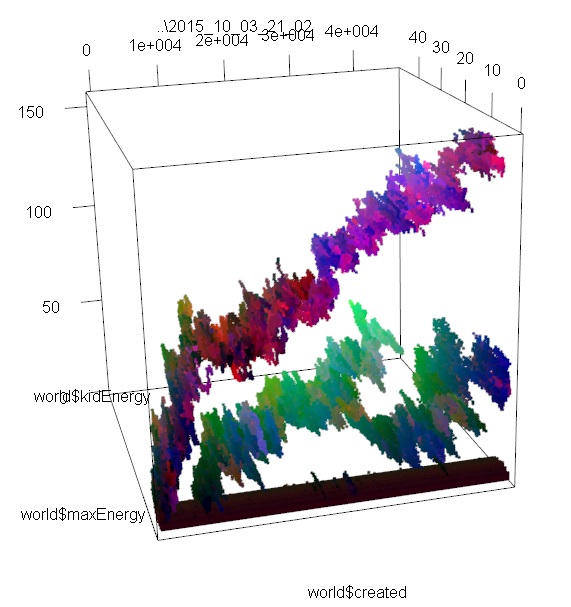
These images represent the distribution of individuals’ properties through time. I encoded 3 properties (don’t remember which) in RGB, so differences in colors also approximately represent different species, or variations in species. In images 1 and 2, you can see 2 or 3 different branches with different ancestors. On these I was only looking at the max amount of energy that each individual can store, and how much of this energy is passed from the parent to its kids. If I had looked instead at speed, or at the number of sensors, we may have seen the branches divide in even smaller branches.
In the 3rd image, we see much more interesting patterns; the lower branch divides clearly in 2 species that have the same original ancestor; then one branch dies out, and the other divides again, and again! To obtain these results, I just doubled the area of the simulation, and maybe cranked up the amount of free energy too (the original one was extremely extremely tiny compared to what is used in Artificial Life usually. Even when doubling the area, I don’t think I ever heard about such a small scale simulation.).
Still, the area was bigger but one important motor of speciation was missing. In real life, species tend to branch out because they become separated by physical obstacles like oceans, mountains, or just distance. To simulate that, I made “mobile areas” of light. Instead of having a fixed square, I had several small areas producing light, and these areas slowly move around. It’s like tiny islands that get separated and sometimes meet each other again, and it looks like this:

Now the species have to follow the light sources, but they can also meet each other and “infect” each other’s islands, competing for resources (or just to eat each other). The trees you get with this are like this:
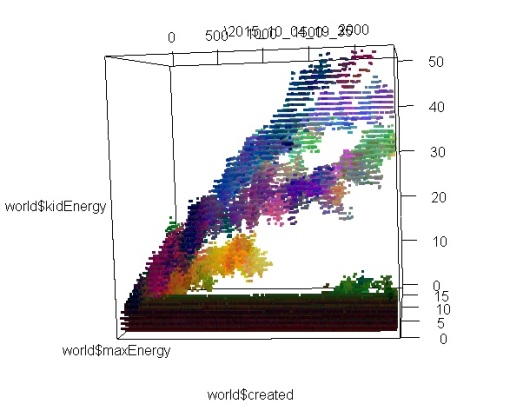
Even more interesting! So many branches! And just looking at the simulation is also loads of fun. Each one is a different story. Sometimes there is drama…

I was rooting for the “smart guys” (fast and with many sensors) above, but they eventually lost the war and went extinct.
What do we take out of that? First, some of the predictions I made in previous posts got realised. The Interface Theory of Perception does allow for a variety of different worlds with their own histories. Additionally, refusing to encode species in the simulation does lead to interesting interactions, and speciation becomes an emergent property of the world. Individuals do not have a property names “species”, or a “species ID” written somewhere. Despite that we don’t end up with a “blob of life” with individuals spread everywhere, we don’t have a “tree of life” clean and straight like in textbooks. It’s more of a beautiful mutant broccoli of life, with blobs and branches. And this sim doesn’t even have sexual reproduction in it! That would make the broccoli even cooler.
The next step in the sim was to implement energy arrays, as I mentioned in an earlier post. I already started and then I kinda forgot. Hopefully I’ll find time to do it!
Conclusion: Did I build an OEE world? Ok, probably not. But I like it and it lived to my expectations.